Location
Mathematics/Psychology : 106
Date & Time
April 10, 2015, 11:00 am – 12:00 pm
Description
Speaker
Dr. Michael Rosenblum
Assistant Professor of Biostatistics
Johns Hopkins Bloomberg School of Public Health.
Title
Optimal Tests of Treatment Effects for the Overall Population and Two Subpopulations in Randomized Trials, using Sparse Linear Programming
Abstract
We propose new, optimal methods for analyzing randomized trials, when it is suspected that treatment effects may differ in two predefined subpopulations. Such subpopulations could be defined by a biomarker or risk factor measured at baseline. The goal is to simultaneously learn which subpopulations benefit from an experimental treatment, while providing strong control of the familywise Type I error rate. We formalize this as a multiple testing problem and show it is computationally infeasible to solve using existing techniques. Our solution involves first transforming the original multiple testing problem into a large, sparse linear program. We then solve this problem using advanced optimization techniques. This general method can solve a variety of multiple testing problems and decision theory problems related to optimal trial design, for which no solution was previously available. Specifically, we construct new multiple testing
procedures that satisfy minimax and Bayes optimality criteria. For a given optimality criterion, our approach yields the optimal tradeoff between power to detect an effect in the overall population versus power to detect effects in subpopulations. We give examples where this tradeoff is a favorable one, in that improvements in power to detect subpopulation treatment effects are possible at relatively little cost in additional sample size. We demonstrate our approach in examples motivated by two randomized trials of new treatments for HIV. This
work is published in the Journal of the American Statistical Association (Theory and Methods), available online here:
Bio:
Michael Rosenblum is an Assistant Professor of Biostatistics at Johns Hopkins Bloomberg School of Public Health. He received his Ph.D. in Applied Math from MIT, followed by a postdoc with Mark van der Laan at the University of California, Berkeley. A major focus of his research is developing improved methods for the design and analysis of randomized trials. Specific goals include (i) developing adaptive trial design methods to determine which subpopulations benefit from
and are harmed by different treatments, and (ii) developing open-source, software tools to make new, proven design and analysis methods widely available and easy to implement.
Tags:
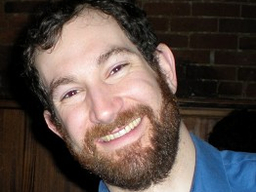