Dr. Alen Alexanderian, NC State University
Location
Mathematics/Psychology : 104
Date & Time
October 7, 2016, 2:00 pm – 3:00 pm
Description
Abstract: When solving an inverse problem, we seek to reconstruct a model parameter, using experimental data observed at a set of sensor locations and using the governing PDEs. The inversion parameter can be a coefficient field appearing in the governing PDEs, a source term, initial condition, or boundary data. Casting the inverse problem in a Bayesian framework, I will discuss the problem of optimizing the location of sensors (at which observational data are collected) to minimize the uncertainty in the parameters estimated by solving the inverse problem. In particular, we can express the uncertainty by the trace of the posterior covariance operator, leading to the Bayesian A-optimal experimental design criterion. Computing optimal experimental designs is particularly challenging for inverse problems governed by computationally expensive PDEs with infinite-dimensional (or, after discretization, high-dimensional) parameters. Focusing on Bayesian linear inverse problems with infinite-dimensional parameters, I will discuss a computational framework for efficient computation of A-optimal sensor placements, which exploits the problem structure and incorporates low-rank approximations, randomized linear algebra, and sparsifying penalty functions. In particular, we find that an optimal design can be computed at a cost, measured in number of forward PDE solves, that is independent of the discretized parameter and sensor dimensions.
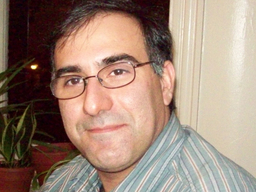