George Mason University
Location
Mathematics/Psychology : 104
Date & Time
February 15, 2019, 2:00 pm – 3:00 pm
Description
Speaker: Tyrus Berry, George Mason University
The theory of kernel density estimation (KDE) has recently been extended to kernel operator estimation (KOE). We introduce notions of pointwise and spectral consistency for operator estimators and explore the bias-variance tradeoff. The variance of these estimators reveals that fixed-bandwidth kernel methods are not consistent on non-compact manifolds (unlike KDE where fixed bandwidth is not a barrier to consistency). Using variable-bandwidth kernels we obtain consistent operator estimators on a large class of non-compact manifolds. These results can be illustrated with simple graph constructions on small data sets, but have far-reaching consequences. Moreover, the bias-variance tradeoff reveals the source of the curse-of-dimensionality and indicates a possible direction towards analyzing high(er)-dimensional data. Finally, for manifolds with boundary we introduce a boundary distance estimator which enables consistent density estimation.
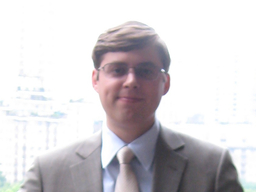