Doctoral Dissertation Defense: Neha Agarwala
Advisors: Drs. Anindya Roy and Junyong Park
Location
Online
Doctoral Dissertation Defense: Neha Agarwala – Online Event
Date & Time
March 15, 2022, 8:00 pm – 9:00 pm
Description
Title: Statistical Inference on High Dimensional Normal Mean Under Linear Inequality Constraints and Efficient Integration of Data in Meta-Analysis
Abstract
In this dissertation, we provide a framework for incorporating linear inequality parameter constraints in estimation and hypothesis testing involving high dimensional normal mean vector. Modern statistical problems often involve such linear inequality constraints on model parameters. Ignoring natural parameter constraints usually results in less efficient statistical procedures. To this end, we define a notion of `sparsity' for such restricted sets using lower-dimensional features. We allow our framework to be flexible so that the number of restrictions may be higher than the number of parameters. We show that the proposed notion of sparsity agrees with the usual notion of sparsity in the unrestricted case and prove the validity of the proposed definition as a measure of sparsity. The proposed sparsity measure allows us to generalize popular priors for sparse vector estimation to the constrained case. Along with Bayesian estimation of the constrained mean, we also consider the classical one-sided normal mean testing problem where the null hypothesis of a zero mean vector is tested against the alternative that all the components are non-negative and at least one is positive. In high dimension, it is unlikely for a single test to perform equally well for dense and sparse parameter configuration. We develop a computationally efficient omnibus test with reasonable power for the entire spectrum of alternatives. Finally, we propose a meta-analysis approach for combining treatment effects across aggregate data (AD) and individual patient data (IPD) under a generalized linear model structure. Often for some studies with AD, the associated IPD may be available, albeit at some extra effort or cost to the analyst. For many different models, design constraints under which the AD estimators are the IPD estimators, and hence fully efficient, are known. For such models, we advocate a selection procedure that chooses AD studies over IPD studies to force least departure from design constraints using the proposed combination method and hence ensures an efficient combined AD and IPD estimator.
Tags:
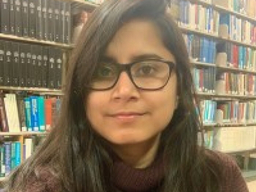