Graduate Students Seminar
Online on Blackboard Collaborate
Location
Online
Date & Time
March 30, 2022, 11:00 am – 12:00 pm
Description
Session Chair: | Neha Agarwala |
Discussant: | Dr. Hye-Won Kang |
Speaker 1: Gaurab Hore
- Title
- FLIP: A Utility Preserving Privacy Mechanism for Time Series
- Abstract
- Adding noise to the data has been the most common method in preserving privacy. That is quite difficult to do in time series data as adding noise to time series data may significantly change the correlation structure, a quantity that is essential to optimal prediction. We propose a privacy mechanism for regularly sampled time series data which preserves utility while providing sufficient privacy guarantees to entity level time series.
Speaker 2: Saeed Damadi
- Title
- Amenable Sparse Network Investigator
- Abstract
- As the optimization problem of pruning a neural network is nonconvex and the strategies are only guaranteed to find local solutions, a good initialization becomes paramount. To this end, we present the Amenable Sparse Network Investigator ASNI algorithm that learns a sparse network whose initialization is compressed. The learned sparse structure found by ASNI is amenable since its corresponding initialization, which is also learned by ASNI, consists of only 2L numbers, where L is the number of layers. Requiring just a few numbers for parameter initialization of the learned sparse network makes the sparse network amenable. The learned initialization set consists of L signed pairs that act as the centroids of parameter values of each layer. These centroids are learned by the ASNI algorithm after only one single round of training. We experimentally show that the learned centroids are sufficient to initialize the nonzero parameters of the learned sparse structure in order to achieve approximately the accuracy of non-sparse network. We also empirically show that in order to learn the centroids, one needs to prune the network globally and gradually. Hence, for parameter pruning we propose a novel strategy based on a sigmoid function that specifies the sparsity percentage across the network globally. Then, pruning is done magnitude-wise and after each epoch of training. We have performed a series of experiments utilizing networks such as ResNets, VGG-style, small convolutional, and fully connected ones on ImageNet, CIFAR10, and MNIST datasets.
Tags:
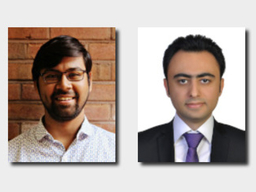