Graduate Students Seminar
Online on Blackboard Collaborate
Location
Online
Date & Time
May 4, 2022, 11:00 am – 12:00 pm
Description
Session Chair: | Alex Sheranko |
Discussant: | Dr. Jinglai Shen |
Speaker 1: Zainab Almutawa
- Title
- Model for Oscillations of Pancreatic Beta Cells by Gap Junction Coupling
- Abstract
- Bursting oscillations in excitable systems reflect multi-timescale dynamics. These oscillations have often been studied in mathematical models by splitting the equations into fast and slow subsystems. Here, we use a simplified model for pancreatic Beta cells. Typically, one treats the slow variables as parameters of the fast subsystem and studies the bifurcation structure of this subsystem. This has key features such as a z-curve and a Hopf bifurcation that gives rise to a branch of periodic spiking solutions. We have extended the theory of bursting of pancreatic Beta-cells from single cells to coupled pairs of cells and how adding a gap junction coupling effect on oscillation.
Speaker 2: Mouhamed Oloude
- Title
- Methods of optimizing the selection of covariates and interations in estimating propensity score
- Abstract
- Randomized experiments are widely used to study the causal effect of an exposure or treatment. In RCTs, since the group exposed to the treatment are randomly selected, observed characteristics, such that age, education, income, are balanced on average, leading to an unbiased estimation of the average treatment effect (ATE). Randomized Clinicals Trials (RCT) are not always feasible for different practical or ethical reasons. One instance is the study of comparison of two methods of surgery, or rare diseases. Observational studies can be a practical tool for studying causal effects in such situations. Rubin and Rosenbaum (1983) proposed an approach for estimating the causal relationship between an exposure and an event in observational studies by simply controlling confounding biases due to the covariates. In this talk, we will explore two methods of selecting the covariates to ensure an effective inference based on an unbiased average causal effect of a treatment. One method is a stepwise procedure that uses logistic regression (log odd ratio) to estimate the unknown parameters of the basic covariates, then adjust the covariates progressively with addition of linear and quadratic terms. Proposed by Kosuke Imai and Marc Ratkovic, the second method is the optimization of the covariate balancing that is attained through the estimation of the propensity score. The model considers the assignment mechanism in estimating the propensity score to prevent a potential misspecification that may occur in the process of estimating the causal effects of a treatment.
Tags:
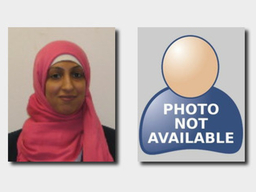