Graduate Students Seminar
Location
Mathematics/Psychology : 103
Date & Time
October 19, 2022, 11:00 am – 12:00 pm
Description
Session Chair: | Ellie Gurvich |
Discussant: | Dr. Baek |
Speaker 1: Ahmet Kaan Aydin
- Title
- A new method for estimating missing correlations in distributed studies
- Abstract
- Space-discretized Finite Difference approximations for the multi-layer Mead-Marcus beam model (PDE) with both hinged and cantilevered boundary conditions are investigated. The PDE model itself describes transverse vibrations for a multi-layer sandwich beam whose outer layers are piezoelectric or elastic, and the constrained layers are viscoelastic. Even though the PDE model (with either type of boundary conditions) is known to be exactly observable with a single observation on its boundary, the discretized models are not able to retain the exact observability uniformly with respect to the mesh parameter. This is mainly due to the loss of the uniform gap among the eigenvalues as the mesh parameter goes to zero. Two techniques are implemented: Direct Fourier Filtering and Order- Reduction. It is shown that the uniform observability is recovered uniformly with respect to the mesh parameter. Recent results and open problems will be discussed, and the Wolfram Demonstrations Projects will be shared with the audience.
Speaker 2: Vahid Andalib
- Title
- State and Parameter Estimation from Partial State Observations in Stochastic Reaction Networks
- Abstract
- In neuroscience, multiple primary/secondary continuous endpoints in a Phase 3 trial have unfortunately not been previously validated. Limited experience and replication of primary/secondary endpoints have led to unexpected trial results leading to a small statistical power. Executing such trials requires considerable time and resources from both the sponsors and trial participants unarguably. Fortunately, many of these unexpected endpoint behaviors can be observed blindly mid-trial. The U.S. Food and Drug Administration (FDA) has clear guidance on adaptive trial designs when the trial adapts to sample size. The FDA, however, has not issued any guidance on adapting the primary endpoint using non-comparative (blinded) data. The FDA has substantial concerns about changing the prespecified primary endpoint mid-trial because of the lack of robust approaches to control the type I error. The Neuroscience Biostatistics group at Genentech company has come up with an approach utilizing the blinded coefficient of variation (CV) for adapting the primary endpoint that controls the type I error under the t-test framework. In this internship project, we extend the t-test blinded CV adaptation to the mixed model with repeated measures (MMRM), and analytically show that it controls type I error.
Tags:
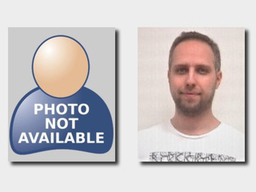