Graduate Students Seminar
Location
Mathematics/Psychology : 103
Date & Time
November 16, 2022, 11:00 am – 12:00 pm
Description
Session Chair: | Lara Scott |
Discussant: | Dr. Webster |
Speaker 1: Jalil Ahmad
- Title
- Neural Net Solvers in Mathematical Epidemiology: A Prediction Model for COVID-19 Cases in Turkey
- Abstract
- In this talk, we first discuss certain mathematical epidemiology models. Then we introduce Mesh Adaptive Direct Search (MADS) as a derivative free optimization technique. We apply this method to neural network models for solving initial value problems. We also present a Residual Network (ResNet) model, parameterized by MADS, for solving these epidemiology problems, with the aim of understanding the spread of COVID-19. Finally, we train our ResNet with finitely observed COVID-19 data that were announced by the Ministry of Health in Turkey; using this training data we make predictions about the spread of the virus. Results suggest that the inconsistent data makes it difficult to train the ResNet, so some data approximation techniques may be helpful in overcoming this issue.
Speaker 2: Weixin Wang
- Title
- A Comparison Between Convolutional and Recurrent Networks on Sequential Data
- Abstract
- For most deep learning practitioners, sequence modeling, such as time series forecasting, is synonymous with recurrent networks. Yet recent results indicate that convolutional architectures can outperform recurrent networks on tasks such as audio synthesis and machine translation. Given a new sequence modeling task or dataset, which architecture should one use? We conduct a systematic evaluation of generic convolutional and recurrent architectures for sequence modeling. The models are evaluated across a broad range of standard tasks that are commonly used to benchmark recurrent networks. Our results indicate that a simple convolutional architecture outperforms canonical recurrent networks such as LSTMs across a diverse range of tasks and datasets, while demonstrating longer effective memory. We conclude that the common association between sequence modeling and recurrent networks should be reconsidered, and convolutional networks should be regarded as a natural starting point for sequence modeling tasks.
Tags:
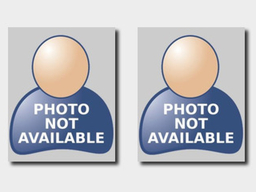