Graduate Students Seminar
Location
Mathematics/Psychology : 103
Date & Time
November 30, 2022, 11:00 am – 12:00 pm
Description
Session Chair: | Bo Liu |
Discussant: | Dr. DoHwan Park |
Speaker 1: Fred Azizi
- Title
- A review of regularized regression methods for high dimensional setting with focus on time-to-event data
- Abstract
- Classical theory for linear regression is well developed. However, in high dimensional settings (when we have more features than observations or more famously when p > n), classical regression models generate undesirable results since they are either infeasible to fit or likely to incur low predictability due to over-fitting. We will review one of the methods of overcoming this issue in penalized models (also known as the regularized model or shrinkage model). We will review some variations of penalized models that are appropriate for analyzing time-to-event data.
Speaker 2: Francesca R. McFadden
- Title
- Unsupervised Machine Learning for Measurement Data
- Abstract
- Utility of Machine learning techniques is predicated on the available [training] data which may be accessed, measured, or simulated. Machine learning techniques utilize the point estimates from a data set to surmise the data category, predict data estimates, and select features for algorithm evaluation. There are two major types of Machine learning algorithms - Supervised versus Unsupervised. Unsupervised techniques are applied to unlabeled data. Clustering is an unsupervised machine learning technique to group similar observations in the data set into a discrete number of clusters. The talk provides an overview of the K-means clustering algorithm. A comparison of performance results across three example distance metrics is provided.
Tags:
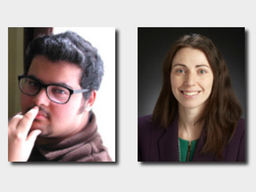