Graduate Students Seminar
Location
Mathematics/Psychology : 106
Date & Time
April 5, 2023, 11:00 am – 12:00 pm
Description
Session Chair: | Weixin Wang |
Discussant: | Dr. DoHwan Park |
Speaker 1: Sidd Roy
- Title
- A Latent Health Process Model for Dynamic Risk Prediction with Application to an HPV study
- Abstract
- Screening programs identify individuals with an elevated risk of diseases. These at-risk individuals may require more frequent screening and collection of additional biomarkers to improve risk assessment at each screening visit. However, standard joint modeling approaches for dynamic risk prediction for these at-risk individuals have several limitations. First, they label individuals who transition back to low-risk status (ex. clearing an infection) as right censored. Furthermore, for non-terminal events, biomarkers collected after the event are often ignored, or biomarker models do not adjust for the event status. We propose to model the health status of at-risk individuals as an underlying stochastic process constrained between two thresholds- an upcrossing representing a transition to low-risk status and a down crossing representing an event. We link the change in the health process to a longitudinal biomarker whose trajectory can change based on the event. Simulations and a real data set show that treating individuals that become risk-free as right censored and ignoring the event's impact on the biomarker trajectory can result in significantly biased risk estimates.
Speaker 2: Saeed Damadi
- Title
- Unifying overparametrized models satisfying the SGC
- Abstract
- Overparameterized machine learning models are capable of fitting the data completely, or memorizing all samples. From the perspective of optimization, this implies achieving a global minimum for a loss function. To analyze these overparameterized models the Strong Growth Condition (SGC) has been a widespread assumption for the convergence analysis of different variants of the Stochastic Gradient Descent (SGD) algorithm. In this presentation we establish a new condition that implies the SGC and show that there is a special class of functions for which this new condition is always satisfied. This class of functions incorporates well-known functions such as mean squared errors, binary cross entropy (logistic loss), and squared hinge loss. Our analysis shows that for this specific class of functions, the constant in the SGC only depends on data, not the function itself. Once this condition holds, we prove that if the mini-batch stochastic gradient is used as the stochastic approximation of gradient, there always exists a fixed step-length (learning rate) for which one can decrease the value of the loss function on average, i.e., the sequence of the expected function values is a suppermartingale sequence..
Tags:
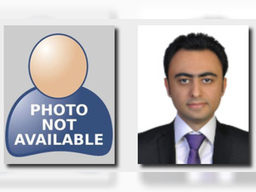