Doctoral Dissertation Defense: Gaurab Hore
Advisors: Drs. Anindya Roy and Tucker McElroy
Location
Mathematics/Psychology : 401
Date & Time
November 8, 2023, 2:30 pm – 4:30 pm
Description
Title: Essays on privacy preserving mechanisms for time series data that maintain utility
Abstract
This dissertation addresses the critical issue of ensuring privacy while preserving data utility in the context of univariate and multivariate time series data. The conventional noise-addition privacy mechanism can distort autocorrelation patterns of time series, thus compromising utility. To overcome this limitation, in a recent paper by McElroy et al. (2023), a mechanism called FLIP has been introduced that uses all-pass filtering to obtain a privatized time series having second-order utility. FLIP has limitations in two key aspects: first, obtaining an all-pass filter becomes significantly more complex in the context of multivariate time series data; and second, it relies on estimation of the spectral density of the time series. To overcome the limitations of FLIP, this dissertation presents two novel approaches. First, we propose a multivariate all-pass (MAP) filtering method, employing an optimization algorithm to achieve the best balance between data utility and privacy protection. A model-agnostic data release mechanism is proposed in the next chapter, which ensures privacy by employing filtering with random coefficients. To preserve data utility, the method releases multiple copies of the time series perturbed using independent random filters. This approach eliminates the need for estimation of the spectral density, making it a more versatile solution for data-producing agencies. The practical performance of the proposed methods is rigorously assessed through numerical studies, including both simulated data and real data sourced from the U.S. Census Bureau’s Quarterly Workforce Indicator (QWI) dataset. By combining the insights from both chapters, this dissertation contributes to the evolving field of privacy mechanisms and data privatization for time series data, offering innovative solutions to the ever-growing challenges faced by data producers and curators.
Tags:
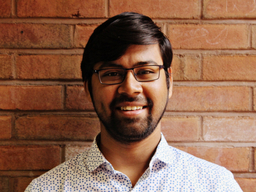