Doctoral Dissertation Defense: Sidd Roy
Advisors: Drs. Anindya Roy and Danping Liu
Location
Mathematics/Psychology : 412
Date & Time
November 20, 2023, 9:30 am – 11:30 am
Description
Title: Flexible Joint Models for Screening Studies
Abstract
In this dissertation, we study and develop statistical methods to jointly analyze longitudinal biomarkers with time to even outcomes motivated by risk assessment in cancer screening. Cancer screening studies collect longitudinal biopsies to allow identification of additional longitudinal biomarker measurements for risk stratification. However, the limited number of biopsies in current studies, screening visit frequency based on risk status, and the need to assess biomarkers useful for early detection of cancer and/or risk prediction present challenges to current joint modeling approaches.
In chapter 2, we develop a dynamic risk prediction approach that can incorporate multiple longitudinal measurements of biomarkers to improve identification of high-risk patients for better clinical management. We propose a joint model to link both a continuous biomarker and a binary biomarker to a time to event precancer outcome using shared random effects. The model uses a discretization of the time scale to allow for closed-form likelihood expressions, allows interval-censored time-to-event outcome due to intermittent clinical visits, incorporates sampling weights to deal with stratified sampling data, and can provide current and future risk estimates that may inform clinical decision-making. Applying the method to longitudinally measured HPV methylation data improves risk stratification for triage of HPV+ women.
In chapter 3, we develop a joint model where the underlying health status for at risk patients is treated as a latent stochastic process constrained between two thresholds where threshold crossing represents transitions to case status or low-risk status. We link the change in the health process to a longitudinal biomarker whose trajectory is allowed to change based on the event. This framework is helpful for cancer screening when biomarkers are used for both risk prediction and early detection and high risk groups is screened more frequently with additional biomarker measurements to better estimate risk. Standard joint model analyses treat individuals that go back to low risk status as right censored and ignore biomarker measurements after the event. Simulations and a real data set show that treating individuals that become risk-free as right censored and ignoring the event's impact on the biomarker trajectory can result in significantly biased risk estimates.
In chapter 4, we compare three common approaches to identify longitudinal biomarkers associated with survival outcomes: joint models, conditional models, and time dependent Cox models. For cancer biomarkers, associations can be acute, meaning longitudinal trajectory may change sharply just before diagnosis or have more long-term associations for risk estimation, such as differences in levels or slopes. Each of the three methods uses a different modeling framework for the joint density of the biomarkers and survival time and thus has different advantages and disadvantages for detecting local and long-term associations. The current project investigates the three approaches' power and type I error under different data generation schemes to motivate further methods development for longitudinal biomarker screening in cancer studies.
Tags:
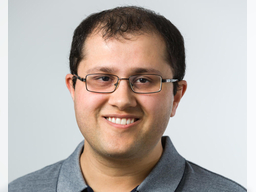