Graduate Students Seminar
Location
Mathematics/Psychology : 103
Date & Time
March 27, 2024, 11:00 am – 12:00 pm
Description
Session Chair: | Naghmeh Akhavan |
Discussant: | ? |
Speaker 1: Lara Scott
- Title
- Chemoattractant Distribution in Biologically Accurate Geometry
- Abstract
- The chemoattractant distribution is known to affect cell migration but its impact in complex geometry has been less well studied. We use Drosophila egg chambers to study cell migration. We import the geometry from images of the Drosophila egg chamber in order to more accurately estimate how the chemoattractant will move through the extracellular space. We create a reaction-diffusion partial differential equation (PDE). The anterior side of the oocyte has a flux condition given by the chemoattractant secretion rate. We use Matlab's finite element PDE solver to see how the chemoattractant will diffuse throughout the extracellular space.
Speaker 2: Vishal Subedi
- Title
- Classifying violent anti-government conflicts in Mexico : A machine learning framework
- Abstract
-
Domestic crime, conflict, and instability present grave challenges to contemporary governments, particularly evident in modern-day Mexico. While strides have been made in forecasting intrastate armed and electoral conflict, these efforts often overlook spatial and temporal correlations, limiting their efficacy. Although deep learning techniques like Convolutional Neural Networks (CNN) excel in predicting conflicts, their black box nature hampers interpretability. To address this, we propose a novel machine learning methodology to accurately predict future anti-government violence in Mexico, identifying key predictors. This aids policymakers in informed decision-making and enables better resource allocation by governments and NGOs. Leveraging political event aggregations from the ICEWS database alongside textual and demographic features, we trained various classical machine learning algorithms and explored CNN + Long Short Term Memory (LSTM) networks. Surprisingly, classical models outperformed black box models. Using Random Forest and Shapley additive values, we identified crucial predictors driving anti-government violence. Our voting classifier, informed by LASSO-derived features, consistently outperformed alternatives, highlighting the significance of factors like homicides, accidents, material conflicts, and citizen sentiment in predicting such conflicts.
Key points: - How to develop a generalised methodology to model spatiotemporal event type political conflict data with binary labels;
Spatial and temporal analysis of the political conflict data;
Identifying predictors that play a crucial role in driving the conflicts.
Tags:
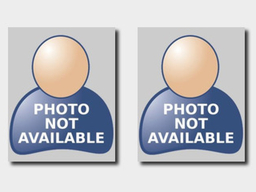