Graduate Students Seminar
Location
Mathematics/Psychology : 103
Date & Time
April 10, 2024, 11:00 am – 12:00 pm
Description
Session Chair: | Yuxin Zhang |
Discussant: | Dr. Thu Nguyen |
Speaker 1: Vahid Andalib
- Title
- High-Dimensional Classifiers With Variable Selection Using Mirror Statistic
- Abstract
- We consider the binary classification problem, in which the data comprises p variables measured on n observations. Significant efforts have been devoted to extending Fisher's linear discriminant analysis (LDA) to address its inadequacy in high-dimensional settings, where p>n. In this paper, we propose a high-dimensional classifier based on LDA, equipped with a variable selection algorithm that utilizes mirror statistic as a univariate test statistic. We construct the mirror statistic using the estimated LDA direction vector to identify the variables contributing the most to classification. In our aim to further improve classification performance, we update the estimated LDA direction vector obtained from the selected variables. This update allows less important direction components a small chance to undergo a sign-flip, while preserving the signs of more useful components with high probability. Extensive simulations and a real-data application demonstrate the competitive performance of our proposed classifier against several existing methods.
Speaker 2: Muhammad Jalil Ahmad
- Title
- Parameter Estimation of ODE Systems with Data Assimilation: A Case Study for Lorenz System
- Abstract
- Models are an important tool for understanding the natural world. Ordinary differential equation models usually include parameters many of which are difficult to estimate. We introduce a novel approach to estimating these parameters by incorporating observable time series data into the estimation. We focus on the Lorenz system as a case study in ODE models since the solutions exhibit a robust variety of behaviors. First, we create a shadow system with a nudging term, a well-known data assimilation technique, that incorporates the observable data and shows that the resulting solutions track the data. Next we minimize a loss function that corresponds to the squared difference between the data and the shadow system over the parameter space. We will illustrate parameter estimation for the Lorenz system using several different numerical optimization techniques. This methodology holds promise for applications where uncertainties in initial conditions and parameter estimates have traditionally posed limitations and provides a robust framework for advancing the utility and reliability of ODE models arising in the natural sciences.
Tags:
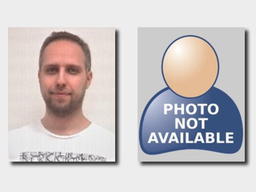