Graduate Students Seminar
Location
Mathematics/Psychology : 103
Date & Time
April 17, 2024, 11:00 am – 12:00 pm
Description
Session Chair: | Alida Schott |
Discussant: | Jinglai Shen |
Speaker 1: Harrison Lewis
- Title
- Processing Vital Signs for Deep Learning: PPG Wave Feature Extraction
- Abstract
- Arterial pulse palpation, that is, direct assessment of the pulse through tactile contact with an artery, has been a staple of medical practitioners longer than recorded history. Over time, examination methods matured, producing a modern analogue known as the photoplethysmogram (PPG). Now, with the advent of sophisticated machine learning methods, the data-driven medical field increasingly looks to researchers to produce powerful predictive models to supplement both the speed and accuracy of a provider's interventions, decreasing the overall workload and freeing their attention for other tasks. We consider the implementation of the Bleeding Risk Index (BRI) framework created by researchers at University of Maryland's medical school. Along the way, we will zoom in to examine the predictive power of the PPG by performing signal analyses to extract and calculate its component features.
Speaker 2: Soumadeep Bhowmick
- Title
- Recent Developments in Inference with Non-Probability Survey Samples
- Abstract
-
Probability sampling has become the gold standard in survey sampling since Neyman's seminal paper (1934). It has been universally applied in research fields such as official statistics, health, informatics, and many others, where surveys are integral to data-driven decisions. In contrast, non-probability sampling, lacking the theoretical foundation and coverage issues, always took the back seat. Recently, the increasing popularity of web-based surveys caused a shift in paradigm as researchers found time and cost-efficient alternatives in non-probability sampling.
In this talk, we will briefly introduce the notions of non-probability sampling and a few approaches that have been proposed recently to leverage the information from the non-probability sample to draw inferences about the population parameter. We will consider a setup where a reference probability sample is also available along with the non-probability sample. For this talk, we will mainly focus on the model-based prediction approach and propensity score-based approach.
Tags:
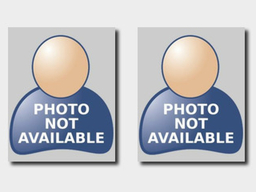