Doctoral Dissertation Defense: Vahid Andalib
Advisor: Dr. Seungchul Baek
Location
Mathematics/Psychology : 412
Date & Time
August 21, 2024, 11:00 am – 1:00 pm
Description
Title: Data-Driven Approaches to Classifier and Variable Selection in High-Dimensional Classification
Abstract
Classification in high dimensions has gained significant attention over the past two decades since Fisher's linear discriminant analysis (LDA) is not optimal in a smaller sample size n comparing the number of variables p, i.e., p>n, which is mostly due to the singularity of the sample covariance matrix. This dissertation proposes two novel data-driven approaches to address the challenges in high-dimensional classification, both building upon Fisher's LDA.
The first approach involves the development of binary classifiers using random partitioning. Rather than modifying how to estimate the sample covariance and sample mean vector in constructing a classifier, we build two types of high-dimensional classifiers using data splitting, i.e., single data splitting (SDS) and multiple data splitting (MDS). We also present a weighted version of the MDS classifier that further improves classification performance. Each of the split data sets has a smaller size of variables compared to the sample size so that LDA is applicable, and classification results can be combined with respect to minimizing the misclassification rate. We provide theoretical justification backing up our methods by comparing misclassification rates with LDA in high dimensions.
The second approach proposes a high-dimensional classifier, which is a two-stage procedure serving variable selection and classification tasks. The variable selection scheme is to select covariates that belong to the discriminative set, and this approach is aimed at obtaining a better classifier, rather than choosing significant variables themselves. In the first stage, we identify discriminative variables by adopting a notion of mirror statistic, proposed recently in the literature, and LDA direction vector obtained from a regularized form of the sample covariance matrix and a James-Stein type estimator for the mean vectors. In the second stage, a new classifier is developed using the selected variables, refined with a modified [image: \epsilon]-greedy algorithm to enhance the LDA direction vector.
Both approaches are extensively validated through simulation studies and real data analysis, including DNA microarray data sets. Our methods demonstrate superior or comparable performance to existing high-dimensional classifiers, offering improved classification accuracy, effective variable selection, and robustness in various scenarios.
This dissertation contributes to the field of high-dimensional statistics by providing novel, theoretically grounded, and effective methods for classification in high-dimensional spaces, with potential applications in genomics, machine learning, and other domains facing the challenges of high-dimensional data analysis.
Tags:
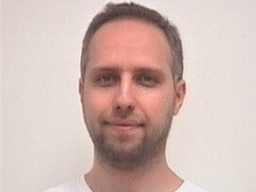