Doctoral Dissertation Defense: Jonathan McHenry
Advisors: Dr. Gobbert and Dr. Neerchal
Location
Sondheim Hall : 203
Date & Time
November 21, 2014, 2:00 pm – 3:00 pm
Description
Title: Parallel Regularized Maximum Likelihood Estimation for Proportional Odds Models
Abstract: Ordinal categorical random variables are random variables which take on values from a finite ordered set of possible values. They often result from qualitative assessments, such as rating a movie from one to five stars. Unlike continuous variables whose values are also ordered, ordinal categories are qualitative, so the distance between them can be unknown.
This work focuses on computational aspects of predicting ordinal categorical random variables. We employ multinomial logistic models as the workhorse of our methodology. Beginning with the proportional odds version of the cumulative logit model, usually called the Proportional Odds Model (POM), we develop a regularized variant of that model. In particular, we study a special case of the generalized L1 regularized POM which we call the Fused Proportional Odds Model (FPOM) because it can result in coefficients whose values are equal, they can be ``fused'' together. FPOM incorporates a penalty term composed of a positive combination of terms: the absolute value of each coefficient, the absolute differences of coefficients, and the absolute differences of consecutive intercepts. This penalty makes sense when there is a notion of adjacency among coefficients, such as occurs in signal processing, and/or when reduction of degrees of freedom is desired, e.g., in the context of high dimensional predictors. The geometry of this optimization, convex with edges and points, can aid finding a sparse solution, which is desirable in some contexts.
As an illustratory example, we apply FPOM to a wine quality assessment dataset. We show, using various measures of prediction accuracy, that our model is competitive with other methods that have been applied to this data. Using a simulation, we show that FPOM can be used for variable selection.
For many modern problems, especially those involving automated data collection, large quantities of data can be produced. Analyzing this data in a reasonable amount of time can be of practical importance. Accordingly, we study the use of parallel computation to speed up FPOM parameter estimation. Using computational nodes with two multi-core processors, we show that enabling parallel computation can result in an order of magnitude speedup on a single computational node.
Abstract: Ordinal categorical random variables are random variables which take on values from a finite ordered set of possible values. They often result from qualitative assessments, such as rating a movie from one to five stars. Unlike continuous variables whose values are also ordered, ordinal categories are qualitative, so the distance between them can be unknown.
This work focuses on computational aspects of predicting ordinal categorical random variables. We employ multinomial logistic models as the workhorse of our methodology. Beginning with the proportional odds version of the cumulative logit model, usually called the Proportional Odds Model (POM), we develop a regularized variant of that model. In particular, we study a special case of the generalized L1 regularized POM which we call the Fused Proportional Odds Model (FPOM) because it can result in coefficients whose values are equal, they can be ``fused'' together. FPOM incorporates a penalty term composed of a positive combination of terms: the absolute value of each coefficient, the absolute differences of coefficients, and the absolute differences of consecutive intercepts. This penalty makes sense when there is a notion of adjacency among coefficients, such as occurs in signal processing, and/or when reduction of degrees of freedom is desired, e.g., in the context of high dimensional predictors. The geometry of this optimization, convex with edges and points, can aid finding a sparse solution, which is desirable in some contexts.
As an illustratory example, we apply FPOM to a wine quality assessment dataset. We show, using various measures of prediction accuracy, that our model is competitive with other methods that have been applied to this data. Using a simulation, we show that FPOM can be used for variable selection.
For many modern problems, especially those involving automated data collection, large quantities of data can be produced. Analyzing this data in a reasonable amount of time can be of practical importance. Accordingly, we study the use of parallel computation to speed up FPOM parameter estimation. Using computational nodes with two multi-core processors, we show that enabling parallel computation can result in an order of magnitude speedup on a single computational node.
Tags:
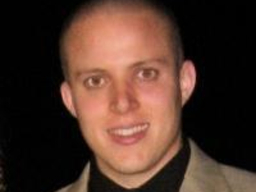