Doctoral Dissertation Defense: Elias Al-Najjar
Advisor: Dr. Adragni
Location
Mathematics/Psychology : 105
Date & Time
July 22, 2015, 2:00 pm – 3:00 pm
Description
Title: Extensions of Cook’s Principal Fitted Components for Sufficient Dimension Reduction
Abstract:
Most methodologies for sufficient dimension reduction (SDR) in regression are limited to continuous predictors, although many data sets do contain both continuous and categorical variables. Application of these methods to regressions that include qualitative predictors such as gender or species may be inappropriate. In part 1 of this thesis we develop an inverse regression based sufficient dimension reduction approach, which can aid regression of a response Y on a set of qualitative predictors W and a vector X of many-valued predictors. In establishing a few straightforward extensions of the principal fitted components (PFC) model, a likelihood-based SDR method, we seek possible sufficient dimension reductions of X that are constrained through the sub-populations established by W. We provide the estimators of the sufficient reduction subspaces and demonstrate how they work through applications.
In part 2 we confront another limitation of the standard principal fitted components model; its assumption that all observations are independent. This assumption is helpful for derivation of a closed-form minimal sufficient dimension reduction subspace via inverse regression. However, consider data recorded on C subjects, where n_j observations of (X,Y) are recorded for each subject j. While the observations from subject to subject could be independent, the within-subject observations are likely dependent. Treating the within-subject observations as independent may not be appropriate, and may impede estimation of the central subspace or make it less efficient. We propose a model for estimation of the central subspace when the observations are dependent within cluster. We use what we call a hierarchical PFC model to estimate the minimal sufficient reduction, which can replace $X$ in a forward population-averaged model. We also further expand this model and develop a way to account for random variation in the central subspace.
Tags:
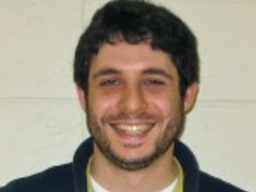