Applied Mathematics Colloquium
James C. Spall, Johns Hopkins University
Location
Mathematics/Psychology : 106
Date & Time
October 9, 2015, 11:30 am – 12:30 pm
Description
James C. Spall
The Johns Hopkins University
Applied Physics Laboratory and Department of Applied Mathematics and Statistics
Abstract: The talk will discuss two key extensions to the basic simultaneous perturbation stochastic approximation (SPSA) algorithm for optimization in a stochastic setting. SPSA in its basic form is in the general family of “first-order” stochastic approximation methods. This talk will present significant extensions that deal with problems at the extremes in some sense: SPSA for adaptive estimation in smooth problems, where we wish to obtain a Hessian estimate, and SPSA-type ideas in fully discrete (combinatorial) problems.
Relative to the smooth case, it is known that a stochastic analogue of the well-known (deterministic) Newton-Raphson algorithm provides an asymptotically optimal or near-optimal form of search for optimization (or root-finding). However, directly determining the required Hessian matrix for optimization has often been difficult or impossible in practice. We review state-of-the-art methods for solving the optimization problem of interest while simultaneously estimating the Hessian matrix.
For the non-smooth case, we discuss the middle point discrete simultaneous perturbation stochastic approximation (DSPSA) algorithm for the optimization of a noisy loss function defined on a multi-dimensional grid of points in Euclidean space. It can be shown that the sequence generated by DSPSA converges to the optimal point under some conditions. Further, the rate of convergence for DSPSA can be analyzed by solving for an upper bound of the mean-squared error of the generated sequence. The error bound allows for a formal comparison of the performance of DSPSA with other discrete algorithms such as the stochastic ruler algorithm and the stochastic comparison algorithm.
Acknowledgment: The discrete work is joint with Dr. Qi Wang of Barclays (former doctoral student at Johns Hopkins University).
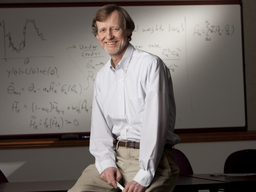