Applied Mathematics Colloquium
Dr. Muruhan Rathinam, UMBC
Location
Mathematics/Psychology : 106
Date & Time
March 25, 2016, 11:30 am – 12:30 pm
Description
Title: Analysis of Monte Carlo estimators for parametric sensitivities in stochastic chemical kinetics
Estimation of parametric sensitivities is an essential ingredient in the process of parameter fitting based on data. For deterministic systems governed by ordinary differential equations (ODEs) the sensitivity defined by a partial derivative of a function of the state f(X(t)) with respect to a parameter c is relatively easy to compute. In contrast, several radically different approaches are available for the computation of the parametric sensitivity defined by the partial derivative of E(f(X(t))) with respect to a parameter c. When the dimension of the state space is large, usually the Monte Carlo
methods are the most efficient and even among these there are several different approaches.
We provide an overview of some of the major Monte Carlo approaches, namely
the Finite Difference (FD), Regularized Pathwise Derivative (PD) and the Girsanov
Transformation (GT). The efficiency of a Monte Carlo approach depends in part on the
estimator having a low variance. It has been numerically observed that in several examples the FD and the RPD methods tend to have lower variance than the GT estimator while the latter has the advantage of being unbiased.
We present a theoretical explanation in terms of system volume asymptotics for the larger variance of the GT approach when compared to the FD methods. We also present an analysis of efficiency of the FD and GT methods in terms of desired error and system volume.
Abstract: We consider parametric sensitivity estimation for well-stirred model of stochastic chemical kinetics which is essential in the modeling of intracellular biochemical reactions. Similar models also appear in epidemiology, predator-prey interactions and other forms of population processes.
Estimation of parametric sensitivities is an essential ingredient in the process of parameter fitting based on data. For deterministic systems governed by ordinary differential equations (ODEs) the sensitivity defined by a partial derivative of a function of the state f(X(t)) with respect to a parameter c is relatively easy to compute. In contrast, several radically different approaches are available for the computation of the parametric sensitivity defined by the partial derivative of E(f(X(t))) with respect to a parameter c. When the dimension of the state space is large, usually the Monte Carlo
methods are the most efficient and even among these there are several different approaches.
We provide an overview of some of the major Monte Carlo approaches, namely
the Finite Difference (FD), Regularized Pathwise Derivative (PD) and the Girsanov
Transformation (GT). The efficiency of a Monte Carlo approach depends in part on the
estimator having a low variance. It has been numerically observed that in several examples the FD and the RPD methods tend to have lower variance than the GT estimator while the latter has the advantage of being unbiased.
We present a theoretical explanation in terms of system volume asymptotics for the larger variance of the GT approach when compared to the FD methods. We also present an analysis of efficiency of the FD and GT methods in terms of desired error and system volume.
Tags:
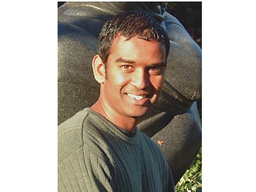