Applied Mathematics Colloquium
Dr. Kody Law, Oak Ridge National Laboratory
Location
Mathematics/Psychology : 104
Date & Time
November 4, 2016, 2:00 pm – 3:00 pm
Description
Title: Data assimilation using multilevel Monte Carlo
Abstract: For half a century computational scientists have been numerically simulating complex systems. Uncertainty is recently becoming a requisite consideration in complex applications which have been classically treated deterministically. This has led to an increasing interest in recent years in uncertainty quantification (UQ). Another recent trend is the explosion of available data. Bayesian inference provides a principled and well-defined approach to the integration of data into an a priori known distribution. The posterior distribution, however, is known only point-wise (possibly with an intractable likelihood) and up to a normalizing constant. This becomes prohibitively expensive when the dimension of the state and/or parameters are high and/or the model is expensive, for example arising as the approximation of a partial differential equation (PDE). If the state is evolving in time and observations are arriving online it becomes even more difficult to iteratively perform inference, known as filtering. Data assimilation [6] refers traditionally to the latter context, however the static smoothing problem arises in so-called re-analysis, where one more carefully analyses a previous fixed data set. In lieu of adhering to the Bayesian probabilistic formulation, one often in practice resorts to more tractable tasks such as tracking the underlying signal (and ideally also some measure of spread). Data assimilation will be introduced, and some connections will be drawn between these perspectives. The rest of the talk will be focused on the development of new algorithms for probing the posterior distribution or an approximation thereof.
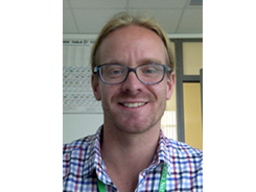