Statistics Colloquium, Dr. Avinash C. Singh
Survey and Data Sciences Division, AIR
Location
Mathematics/Psychology : 104
Date & Time
November 18, 2016, 11:00 am – 12:00 pm
Description
Title: Estimation from Purposive Samples with Probability Sample Supplements but without Any Data on the Study Variable
Abstract:
There is great interest among practitioners in making inferences about characteristics of a study variable in a target population using a purposive (or nonprobability sample) without launching costly new or redesigned probability surveys. We consider a special practical scenario where an extant probability sample already exists that is representative of the target population but does not have information about the study variable. Such a problem arises in many applications such as in the context of making inferences about detailed healthy behavior characteristics using the National Health Interview Survey (NHIS) (which does not collect such information due to response burden already imposed by the current NHIS interview) with the help of an opt-in internet panel survey which collects the required detailed information. Other examples might include the problem of generalizability of randomized trials where an auxiliary probability supplement is available but without any data on the treatment under consideration.
For the above problem, use of propensity score modeling to obtain sample inclusion propensity weights for the purposive sample by treating the purposive sample as the treatment group and the probability supplement as the control may be questionable because the domain of units corresponding to the purposive sample is likely to overlap with the probability supplement sample. Moreover, parameter estimates for the model may be subject to selection bias. We propose an alternative solution termed Model-Over-Design (MOD) integration which is based on an integration of the two fundamental approaches of design-based and model-based estimation in survey sampling. It is assumed that the suitable covariates needed for a linear prediction model are available for both the purposive and probability samples. Also the purposive sample is sufficiently diverse that it includes units with covariate values similar to the probability supplement. If not, then the method can be used for inference about the corresponding subpopulation or domain.
The proposed method requires a design-weighted estimate of the regression model parameters as in the commonly used GREG (generalized regression, Särndal, 1980) estimator in survey sampling which is computed after imputing the study variable in the probability supplement using the purposive sample as a donor dataset. The method of predictive mean matching is used for this purpose where the prediction scores needed for matching are computed iteratively using the design-weighted regression from the probability supplement. The initial values for this iterative procedure are obtained by using the unweighted estimates of regression parameters from the purposive sample. If the purposive sample is sufficiently diverse in terms of covering regions of the covariate values, and the estimated regression parameter estimates converge regardless of the true values in the limit, the synthetic part of the GREG estimator provides a robust estimator because the weighted estimate of the total model error becomes zero in the presence of an intercept term—an important property of GREG. This synthetic estimate is improved by adding a model error correction term given by the unweighted model errors obtained from the seen units in the purposive sample as in the model-based estimator PRED (denoting prediction) of Royall (1972, 1976). The resulting estimator defines the proposed MOD integration estimator. The usual replication methods in survey sampling can be used to obtain variance estimates. Results based on a limited empirical study will be presented to compare main estimators.
Joint work with Cong Ye
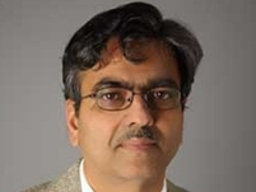