Doctoral Dissertation Defense: Zois Boukouvalas
Advisor: Dr. Tulay Adali
Location
Information Technology/Engineering : 325B
Date & Time
May 24, 2017, 1:30 pm – 3:30 pm
Description
Title: Development of ICA and IVA Algorithms with Application to Medical Image Analysis
Abstract
Blind source separation (BSS) is an active area of research due to its applicability to a variety of problems, especially when there is a little known about the observed data. Applications where BSS has been successfully utilized include the analysis of medical imaging data, such as functional magnetic resonance imaging (fMRI) data, detection of specific targets in video sequences or multi-spectral remote sensing data, among many others.
Independent component analysis (ICA) is a widely used BSS method that can uniquely achieve source recovery, subject to only scaling and permutation ambiguities, through the assumption of statistical independence on the part of the latent sources. Independent vector analysis (IVA) extends the applicability of ICA by jointly decomposing multiple datasets through the exploitation of the dependencies across datasets.
Though both ICA and IVA algorithms cast in the maximum likelihood (ML) framework enable the use of all available statistical information---forms of diversity---in reality, they often deviate from their theoretical optimality properties due to improper estimation of the probability density function (PDF). This motivates the development of flexible ICA and IVA algorithms that closely adhere to the underlying statistical description of the data. Although it is attractive to let the data ''speak'' and hence minimize the assumptions, important prior information about the data, such as sparsity, is usually available. If incorporated into the ICA model, use of this additional information can relax the independence assumption, resulting in an improvement in the overall separation performance. Therefore, the development of a unified mathematical framework that can take into account both statistical independence and sparsity is of great interest.
In this dissertation, we first introduce a flexible ICA algorithm that uses an effective PDF estimator to accurately capture the underlying statistical properties of the data, yielding superior separation performance and maintaining the desirable optimality of ML estimation. We then discuss several techniques to accurately estimate the parameters of the multivariate generalized Gaussian distribution, and how to integrate them into the IVA model and derive a class of flexible IVA algorithms that take both second-order statistics and higher-order statistics into account. Finally, we provide a mathematical framework that enables direct control over the influence of statistical independence and sparsity, and use this framework to develop an effective ICA algorithm that can jointly exploit these two forms of diversity. Hence, by increasing the flexibility of ICA and IVA algorithms and by enriching the models through the incorporation of reliable priors, we enhance the capabilities of ICA and IVA and, thus, enable their application to many problems.
We demonstrate the effectiveness of the proposed ICA and IVA algorithms using numerical examples as well as fMRI-like data.
Abstract
Blind source separation (BSS) is an active area of research due to its applicability to a variety of problems, especially when there is a little known about the observed data. Applications where BSS has been successfully utilized include the analysis of medical imaging data, such as functional magnetic resonance imaging (fMRI) data, detection of specific targets in video sequences or multi-spectral remote sensing data, among many others.
Independent component analysis (ICA) is a widely used BSS method that can uniquely achieve source recovery, subject to only scaling and permutation ambiguities, through the assumption of statistical independence on the part of the latent sources. Independent vector analysis (IVA) extends the applicability of ICA by jointly decomposing multiple datasets through the exploitation of the dependencies across datasets.
Though both ICA and IVA algorithms cast in the maximum likelihood (ML) framework enable the use of all available statistical information---forms of diversity---in reality, they often deviate from their theoretical optimality properties due to improper estimation of the probability density function (PDF). This motivates the development of flexible ICA and IVA algorithms that closely adhere to the underlying statistical description of the data. Although it is attractive to let the data ''speak'' and hence minimize the assumptions, important prior information about the data, such as sparsity, is usually available. If incorporated into the ICA model, use of this additional information can relax the independence assumption, resulting in an improvement in the overall separation performance. Therefore, the development of a unified mathematical framework that can take into account both statistical independence and sparsity is of great interest.
In this dissertation, we first introduce a flexible ICA algorithm that uses an effective PDF estimator to accurately capture the underlying statistical properties of the data, yielding superior separation performance and maintaining the desirable optimality of ML estimation. We then discuss several techniques to accurately estimate the parameters of the multivariate generalized Gaussian distribution, and how to integrate them into the IVA model and derive a class of flexible IVA algorithms that take both second-order statistics and higher-order statistics into account. Finally, we provide a mathematical framework that enables direct control over the influence of statistical independence and sparsity, and use this framework to develop an effective ICA algorithm that can jointly exploit these two forms of diversity. Hence, by increasing the flexibility of ICA and IVA algorithms and by enriching the models through the incorporation of reliable priors, we enhance the capabilities of ICA and IVA and, thus, enable their application to many problems.
We demonstrate the effectiveness of the proposed ICA and IVA algorithms using numerical examples as well as fMRI-like data.
Tags:
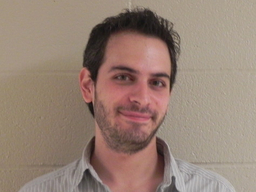