Statistics Colloquium: Dr. Martin Slawski
George Mason University
Location
On Campus : MP401
Date & Time
September 15, 2017, 11:00 am – 12:00 pm
Description
Title: On the use of Random Projections for Dimension Reduction in Linear Regression
Abstract: Principal Components Regression (PCR) is a traditional tool for dimension reduction in linear regression that has been both criticized and defended. One concern about PCR is that obtaining the leading principal components tends to be computationally demanding for large data sets. While Random Projections (RPs) do not possess the optimality properties of the projection onto the leading principal subspace, they are computationally appealing and hence have become increasingly popular in recent years. In this talk, we present an analysis showing that the dimension reduction offered by RPs achieves a prediction error in subsequent regression close to that of PCR, at the expense of requiring a slightly large number of RPs than PCs.
Longitudinal dyadic data with missing data is difficult to analyze due to the complicated inter-and outer correlations within and between dyads, as well as non-ignorable missing data. In this talk, I will introduce 1) a Bayesian mixed effects hybrid model to analyze longitudinal data with non-ignorable dropouts and 2)a Bayesian shared parameter model to analyze longitudinal dyadic data with non-ignorable intermittent dropouts. I factorize the joint distribution of the measurement and dropout processes into three components where the two approaches use different factorizations. The two proposed models account for the dyadic interplay using the concept of actor and partner effects as well as dyad-specific random effects. We evaluate the performance of the proposed methods using a simulation study, and apply our method to longitudinal dyadic datasets that arose from 1) a prostate cancer trial and 2) a metastatic breast cancer study. Through a series of sensitivity analyses, we can provide validity of the proposed model.
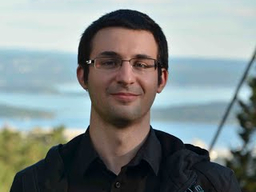