Doctoral Dissertation Defense: Sai Popuri
Advisor: Dr. Nagaraj Neerchal
Location
Sherman Hall : 148C
Date & Time
September 28, 2017, 8:30 am – 10:30 am
Description
Title: Prediction Methods For Semi-Continuous Data with Applications in Climate Science
Abstract
Semi-continuous random variables have discrete and continuous components with support on a set of discrete points and a subset on the real line. Daily precipitation (rainfall) data is an example of such a random variable with a point mass at zero and a continuous distribution on the positive real line. Semi-continuous data arise in various applications ranging from Climate Science to Economics. This dissertation includes both methodological approaches as well as applications. We illustrate our approaches using the precipitation data from MIROC5, a widely used climate model, as a predictor to predict observed precipitation in the Missouri River Basin (MRB).
In this dissertation, we consider the problem of obtaining semi-continuous predictions for semi-continuous data. This dissertation is divided into two parts. In the first part, we begin with a brief review of some inferential aspects of the semi-continuous distributions. Subsequently, we consider the problems of testing whether a sample of semi-continuous data is from a specified distribution and testing for a given restriction in the parameters of the density function. We propose a bootstrap test that is more robust and simpler. Simulation studies show that the test performs better than the three classical large sample tests: Likelihood Ratio (LR), Score, and Wald tests in terms of size and power. We also derive the posterior predictive distributions for semi-continuous data and compare them with the frequentist plug-in (also known as estimative) distributions. It turns out that for a two-part gamma distribution, the posterior predictive distribution with an empirical bayes prior performs better than the corresponding plug-in distribution for a range of parameter values in terms of Kullback-Leibler loss. We propose entropy based methods to approximate these posterior predictive distributions, which are sometimes intractable.
In the second part we present several prediction methods for semi-continuous data in a regression context. We propose a two-step Expectation-Maximization (EM) like method for the daily precipitation data at a location in the MRB. In the first step, the zero values in the time series data are treated as “missing” and are imputed using an Autoregressive (AR) model fitted to the positive data in an iterative fashion. In the second step, a lagged regression model is fitted using the time series data on daily precipitation provided by MIROC5 as a covariate. Predictions from this model show significant improvement over a Bayesian state-space model fitted to the same data.
We end the dissertation with an application of a sufficient dimension reduction technique called Sliced Inverse Regression (SIR) and Nadaraya-Watson prediction, suitably adapted to semi-continuous data, to the spatio-temporal daily precipitation data in the MRB region. Various aspects of the method, including parallel implementation, are discussed.
Abstract
Semi-continuous random variables have discrete and continuous components with support on a set of discrete points and a subset on the real line. Daily precipitation (rainfall) data is an example of such a random variable with a point mass at zero and a continuous distribution on the positive real line. Semi-continuous data arise in various applications ranging from Climate Science to Economics. This dissertation includes both methodological approaches as well as applications. We illustrate our approaches using the precipitation data from MIROC5, a widely used climate model, as a predictor to predict observed precipitation in the Missouri River Basin (MRB).
In this dissertation, we consider the problem of obtaining semi-continuous predictions for semi-continuous data. This dissertation is divided into two parts. In the first part, we begin with a brief review of some inferential aspects of the semi-continuous distributions. Subsequently, we consider the problems of testing whether a sample of semi-continuous data is from a specified distribution and testing for a given restriction in the parameters of the density function. We propose a bootstrap test that is more robust and simpler. Simulation studies show that the test performs better than the three classical large sample tests: Likelihood Ratio (LR), Score, and Wald tests in terms of size and power. We also derive the posterior predictive distributions for semi-continuous data and compare them with the frequentist plug-in (also known as estimative) distributions. It turns out that for a two-part gamma distribution, the posterior predictive distribution with an empirical bayes prior performs better than the corresponding plug-in distribution for a range of parameter values in terms of Kullback-Leibler loss. We propose entropy based methods to approximate these posterior predictive distributions, which are sometimes intractable.
In the second part we present several prediction methods for semi-continuous data in a regression context. We propose a two-step Expectation-Maximization (EM) like method for the daily precipitation data at a location in the MRB. In the first step, the zero values in the time series data are treated as “missing” and are imputed using an Autoregressive (AR) model fitted to the positive data in an iterative fashion. In the second step, a lagged regression model is fitted using the time series data on daily precipitation provided by MIROC5 as a covariate. Predictions from this model show significant improvement over a Bayesian state-space model fitted to the same data.
We end the dissertation with an application of a sufficient dimension reduction technique called Sliced Inverse Regression (SIR) and Nadaraya-Watson prediction, suitably adapted to semi-continuous data, to the spatio-temporal daily precipitation data in the MRB region. Various aspects of the method, including parallel implementation, are discussed.
Tags:
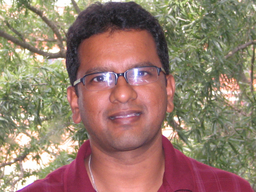