Statistics Colloquium: Dr. Yehenew Kifle
University of Limpopo
Location
On Campus : Sond110
Date & Time
February 23, 2018, 11:00 am – 12:00 pm
Description
Title: Assessing the effect of distance from a dam on time to malaria, with distance confounded with the clustering structure
Abstract: Malaria remains an important disease in terms of morbidity and mortality in many developing countries. Around hydro-electric dams, this risk might even increase due to the large water bodies available to the Anopheles mosquito which functions as a vector for the disease. During two years, time to malaria was followed up on a weekly basis around one of the largest hydro-electric dams in Ethiopia. The households are located at different distances from the dam, which are clustered into 16 villages. The aim of this paper is to study the effect of the distance from the dam on malaria incidence.
Different standard techniques in survival analysis exist to model such clustered survival data, including the marginal, fixed effects, stratified and frailty models. These time to malaria data have certain characteristics that makes the marginal and conditional approaches lead to quite diverse effects. The observed differences in our particular setting are due to the fact that the covariate of interest in the dataset, distance from the dam, is highly confounded with the clustering process, i.e., the village.
Different models that cope with clustering in survival data leads to contradictory results when the covariate of interest is confounded to a large extent with the clustering mechanism. The frailty model is often considered the standard model for clustered survival data. The frailty model estimate is a weighted combination of the within and between village estimate of the distance effect. Such a weighted combination, however, makes only sense if the same relationship holds between and within clusters (village). This assumption, however, is questionable for the type of dataset that is considered in this study. Therefore, in such situation, we advise to split covariates into two orthogonal covariates, one referring to the covariate effect between clusters, and another referring to the covariate effect within clusters.
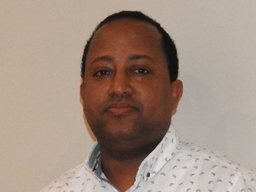