Statistics Colloquium: Dr. Mathangi Gopalakrishnan
University of Maryland-Baltimore County
Location
On Campus : Sond110
Date & Time
February 9, 2018, 11:00 am – 12:00 pm
Description
Title: Model Informed Bayesian Forecasting in Clinical Practice: Acute Acetaminophen Overdose Case Study
Abstract: Model informed therapeutic decision-making tools are being increasingly used in clinical practice for better patient-specific health care outcomes. This talk will demonstrate how pharmaco-statistical model informed Bayesian forecasting was used to predict the need for antidote in acute acetaminophen (APAP) overdose patients.
APAP overdose is the leading cause of acute liver injury in the United States. The current clinical practice is to measure plasma acetaminophen concentrations (PACs) after 4 hours of ingestion and use Rumack-Mathew (RM) Nomogram to decide on administration of an antidote. Prior studies of acute APAP overdoses found that the nomogram failed to accurately predict the need for the antidote in certain patients.
In the current research presented in this talk, a pharmaco-statistical model (non-linear mixed effect model) was developed to describe the APAP concentrations over time following overdose and adjusted for patient-specific prognostic factors. The developed model and the parameters were used as priors for Bayesian forecasting of individual APAP concentration-time profile when limited PACs were available including PACs before 4 hours. The Bayesian forecasted APAP profile demonstrated acceptable sensitivity and negative predictive value in predicting the need for the antidote when compared with the RM nomogram. The model informed Bayesian forecasting tool provided a platform that can potentially be used for early antidote administration decisions (< 4 hours). A prototype R Shiny application implementing the forecasting tool has also been developed.
Tags:
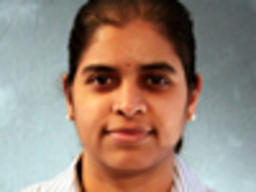