Doctoral Dissertation Defense: Gregory Haber
Advisor: Dr. Yaakov Malinovsky
Location
Mathematics/Psychology : 010
Date & Time
April 18, 2018, 4:00 pm – 6:00 pm
Description
Title: Problems in group testing estimation and design
Abstract
Group testing estimation, which includes any estimation procedure in which units are tested in pools rather than individually, has been an active area of research in the statistical literature for over 70 years. The effective use of such procedures has been shown to lead to large reductions in terms of mean square error (MSE), resulting in more accurate estimates, or allowing for fewer tests to be carried out. Despite these benefits, previous work has relied heavily on large sample methods to establish results. In practice, however, group testing problems will usually involve very small sample sizes so that such methods may be inappropriate. In this dissertation we explore several problems related to group testing estimation and design based on small sample methods.
The first problem we consider is the construction of unbiased estimators. While the standard binomial model does not yield an unbiased estimator, we give a construction based on an inverse binomial model which samples until a fixed number of negative pools are observed. This is extended to include cases where misclassification errors are present, and we show that, while an unbiased estimator can be constructed, it is improper, yielding values outside the parameter space. This is extended to the entire class of binomial sampling plans when misclassification is present, showing that no proper unbiased estimator exists in this broad class. These ideas are extended again to the case of multinomial sampling, where we show that under any sampling plan it is impossible to find a proper unbiased estimator, even without misclassification.
The next problem we consider is the estimation of two diseases simultaneously using group testing methods. No closed form MLE exists in this case, and numerical methods are difficult due to a high frequency of boundary estimates. We propose an EM algorithm based estimator and provide proofs of convergence, even on the boundary of the parameter space. Several closed form alternatives are also provided, primarily with the aim of bias reduction.
The final problem we consider is that of choosing the group size for experiments when only a small number of tests can be carried out. Previous methods have relied heavily on good prior knowledge of the parameter value to be estimated, with the needed accuracy decreasing with the sample size. We propose simple random walk based adaptive procedures which minimize the need for such prior information. These designs are shown numerically to outperform the large sample based methods previously found in the literature. These methods are extended to the case when misclassification errors are present, with similar results.
Abstract
Group testing estimation, which includes any estimation procedure in which units are tested in pools rather than individually, has been an active area of research in the statistical literature for over 70 years. The effective use of such procedures has been shown to lead to large reductions in terms of mean square error (MSE), resulting in more accurate estimates, or allowing for fewer tests to be carried out. Despite these benefits, previous work has relied heavily on large sample methods to establish results. In practice, however, group testing problems will usually involve very small sample sizes so that such methods may be inappropriate. In this dissertation we explore several problems related to group testing estimation and design based on small sample methods.
The first problem we consider is the construction of unbiased estimators. While the standard binomial model does not yield an unbiased estimator, we give a construction based on an inverse binomial model which samples until a fixed number of negative pools are observed. This is extended to include cases where misclassification errors are present, and we show that, while an unbiased estimator can be constructed, it is improper, yielding values outside the parameter space. This is extended to the entire class of binomial sampling plans when misclassification is present, showing that no proper unbiased estimator exists in this broad class. These ideas are extended again to the case of multinomial sampling, where we show that under any sampling plan it is impossible to find a proper unbiased estimator, even without misclassification.
The next problem we consider is the estimation of two diseases simultaneously using group testing methods. No closed form MLE exists in this case, and numerical methods are difficult due to a high frequency of boundary estimates. We propose an EM algorithm based estimator and provide proofs of convergence, even on the boundary of the parameter space. Several closed form alternatives are also provided, primarily with the aim of bias reduction.
The final problem we consider is that of choosing the group size for experiments when only a small number of tests can be carried out. Previous methods have relied heavily on good prior knowledge of the parameter value to be estimated, with the needed accuracy decreasing with the sample size. We propose simple random walk based adaptive procedures which minimize the need for such prior information. These designs are shown numerically to outperform the large sample based methods previously found in the literature. These methods are extended to the case when misclassification errors are present, with similar results.
Tags:
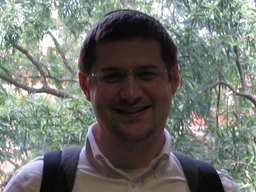