Doctoral Dissertation Defense: Aijun Yu
Advisor: Dr. Anindya Roy
Location
Mathematics/Psychology : 401
Date & Time
July 20, 2018, 10:00 am – 11:00 am
Description
Title: Efficient Designs for Cyclic Data Generated by Multivariate Harmonic Mixed Models
Abstract
The primary focus of this dissertation is to design efficient data collection schemes (including pooling bio-specimens, subsampling and combining laboratory and monitor data) for longitudinal studies, particularly in medical fields where repeated cyclical patterns over time are expected in the model response. Motivation of the work comes from the need for efficient designs in the expensive medical studies where collection of data at regular frequency may not be feasible due to budget constraints.
Throughout the dissertation we use multivariate mixed-effects exponential harmonic models to mimic the cyclical evolution of biomarkers and individual variation. We propose to optimize objective criteria related to efficiency of parameter estimation, such as D-optimality, with respect to pooling and sampling design. Due to analytical intractability, we mostly rely on numerical optimization to devise sensible schemes for finding effective study designs. We apply the optimal design to the BioCycle data.
We propose a combined model to use both monitor and laboratory data to improve the efficiency of the design. The laboratory measurements are more accurate than the monitor ones. Because the accuracy of the laboratory measurements is higher than that of the monitor measurements, when we combine the monitor and laboratory measurements, the efficiency is improved compared to using just monitor data. The efficiency of optimal design based on combined measurements close to that of designs with similar cost and using only laboratory measurements, and much higher than those using equally spaced laboratory measurements. We apply the combined design to the EAGeR study. The combined design improves the efficiency compared to using only laboratory measurements for the luteinizing hormone (LH).
For designs using pooled bio-specimens, we add a novel aspect by incorporating a fatigue model to the data preparation process. We explicitly model the heteroscedasticity of the error of the pooling volume.
Abstract
The primary focus of this dissertation is to design efficient data collection schemes (including pooling bio-specimens, subsampling and combining laboratory and monitor data) for longitudinal studies, particularly in medical fields where repeated cyclical patterns over time are expected in the model response. Motivation of the work comes from the need for efficient designs in the expensive medical studies where collection of data at regular frequency may not be feasible due to budget constraints.
Throughout the dissertation we use multivariate mixed-effects exponential harmonic models to mimic the cyclical evolution of biomarkers and individual variation. We propose to optimize objective criteria related to efficiency of parameter estimation, such as D-optimality, with respect to pooling and sampling design. Due to analytical intractability, we mostly rely on numerical optimization to devise sensible schemes for finding effective study designs. We apply the optimal design to the BioCycle data.
We propose a combined model to use both monitor and laboratory data to improve the efficiency of the design. The laboratory measurements are more accurate than the monitor ones. Because the accuracy of the laboratory measurements is higher than that of the monitor measurements, when we combine the monitor and laboratory measurements, the efficiency is improved compared to using just monitor data. The efficiency of optimal design based on combined measurements close to that of designs with similar cost and using only laboratory measurements, and much higher than those using equally spaced laboratory measurements. We apply the combined design to the EAGeR study. The combined design improves the efficiency compared to using only laboratory measurements for the luteinizing hormone (LH).
For designs using pooled bio-specimens, we add a novel aspect by incorporating a fatigue model to the data preparation process. We explicitly model the heteroscedasticity of the error of the pooling volume.
Tags:
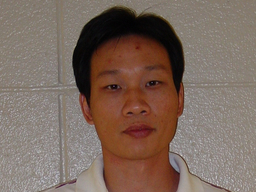