Differential Equations Seminar: Dr. Akwum Onwunta
UMCP
Location
Mathematics/Psychology : 401
Date & Time
October 22, 2018, 11:00 am – 12:00 pm
Description
Title: Fast solvers for PDE-constrained optimization under uncertainty
Abstract: Optimization problems constrained by deterministic steady-state partial differential equations (PDEs) are computationally challenging. This is even more so if the constraints are deterministic unsteady PDEs since one would then need to solve a system of PDEs coupled globally in time and space, and time-stepping methods quickly reach their limitations due to the enormous demand for storage [5]. Yet, more challenging than the aforementioned are problems constrained by unsteady PDEs involving (countably many) parametric or uncertain inputs. A viable solution approach to optimization problems with stochastic constraints employs the spectral stochastic Galerkin finite element method (SGFEM). However, the SGFEM often leads to the so-called curse of dimensionality, in the sense that it results in prohibitively high dimensional linear systems with tensor product structure [1, 2, 4]. Moreover, a typical model for an optimal control problem with stochastic inputs (OCPS) will usually be used for the quantification of the statistics of the system response – a task that could in turn result in additional enormous computational expense. It is worth pursuing computationally efficient ways to simulate OCPS using SGFEMs since the Galerkin approximation yields the best approximation with respect to the energy norm, as well as a favorable framework for error estimation [3]. In this talk, we consider two prototypical model OCPS and discretize them with SGFEM. We exploit the underlying mathematical structure of the discretized systems at the heart of the optimization routine to derive and analyze low-rank iterative solvers and robust block- diagonal preconditioners for solving the resulting stochastic Galerkin systems. The developed solvers are quite efficient in the reduction of temporal and storage requirements of the high-dimensional linear systems [1, 2]. Finally, we illustrate the effectiveness of our solvers with numerical experiments.
REFERENCES
[1] P. Benner, S. Dolgov, A. Onwunta and M. Stoll, Low-rank solvers for unsteady Stokes-Brinkman optimal control problem with random data, Computer Methods in Applied Mechanics and Engineering, 304, pp. 26 – 54, 2016.
[2] P. Benner, A. Onwunta and M. Stoll, Block-diagonal preconditioning for optimal control problems constrained by PDEs with uncertain inputs, SIAM Journal on Matrix Analysis and Applications, 37 (2), pp. 491 – 518, 2016.
[3] A. Bespalov and C. E. Powell and D. Silvester, Energy norm a posteriori error estimation for parametric operator equa- tions. SIAM Journal on Scientific Computing, 36 (2), pp. A339 – A363, 2013.
[4] E. Rosseel and G. N. Wells, Optimal control with stochastic PDE constraints and uncertain controls, Computer Methods in Applied Mechanics and Engineering, 213-216, pp. 152 – 167, 2012.
[5] M. Stoll and T. Breiten, A low-rank in time approach to PDE-constrained optimization, SIAM Journal on Scientific Computing, 37 (1), pp. B1 – B29, 2015.
Tags:
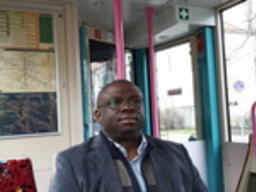