Doctoral Dissertation Defense: Wenxin Lu
Advisor: Dr. Yi Huang
Location
Mathematics/Psychology : 102
Date & Time
August 16, 2019, 9:30 am – 11:30 am
Description
Title: Analysis of Longitudinal Interval Reported Binary Recurrent Event Data & Statistical Model for Subgroup Identification in Enrichment Design
Abstract
Abstract
This thesis contains two research projects. The first thesis project investigates the analytical methods for longitudinal interval reported binary recurrent event data. This project is motivated by the post hip fracture infection project using Baltimore Hip studies (BHS). The infection related outcomes were collected longitudinally using questionnaire items like "Since the last time we spoke in (Provide Month), have you ever had fever?". Even though a subject could miss a few scheduled visits, such questionnaire design only captured the available longitudinal fever data across available visiting months, where the missing visits were skipped and merged into the reporting interval. Another feature is that the recurrent events of interest was observed dichotomously - only the binary status of occurrence in the reporting interval, without frequency counts information nor when they re-occur in this interval. Even though the literature on longitudinal binary data are quite comprehensive, the longitudinal models accounting for interval reported and binary recurrent event features are quite limited. We proposed two longitudinal models in this project, where discrete survival modeling technique and Poisson process are used to account for interval censored reporting system between longitudinal visits and binary nature of recurrent events outcomes. The intensity function follows Cox regression structure allowing for both subject's baseline characteristics and time-varying covariates, which leads to varying intensities over longitudinal visits but fixed intensity within each reporting interval. Simulation studies are used to compare the proposed models vs. standard longitudinal models with logit link to see how well they will capture the significant cross-sectional and longitudinal effects, especially with or without considering interval reporting nature, with or without time-varying covariates, and some other sensitivity analyses to model mis-specifications. Various simulation studies confirm the great performance of the proposed GLMM model with comp(log-log) link. Then, I implemented both the proposed and standard methods on the infection project using BHS data. Out of all 4 models, only the proposed GLMM model with comp(log-log) link detected the statistically significant monthly increasing trend of hazard of infection re-occurrence during the first year hip fracture post-surgery recovery time. And, all models confirmed no sex difference in various measures of infection re-occurrence risks on average over time during the first year follow ups.
The second research project is on the statistical model for subgroup identification in enriched clinical trial design. Enrichment designs have been widely used in randomized clinical trials (RCT) for years across pharmaceutical industry and academia, because such designs are often more efficient, such as smaller sample size, shortened development time, and reduced cost. The enrichment design strategies can be summarized into three categories, which are well documented in the FDA guidance as the prospective use of any patient's characteristics to select a target study sub-population (called "subgroup") smartly, so that the drug effects (if one is in fact present) are easier and clearer to be detected than the unselected population. Based on the information from the phase II RCTs and prior scientific knowledge from historical literature and other studies, the current popular practice of enrichment design strategy is to use individual indicator variable as the criteria for subgroup identification. However, this strategy becomes infeasible when the number of associated variables or criteria increases. Thus, in this thesis project, we build a subgroup selection model using many patients' characteristics, which could be estimated by outcome regression model, inverse probability weighted estimator (IPWE), and doubly robust inverse probability weighted estimator (DRIPWE). The purpose is to facilitate the subgroup identification and find the ideal target sub-population for phase III participants, making phase III RCT more efficient. Simulation studies are used to compare the three proposed methods on building the optimal subgroup selection model and demonstrate the importance to include as many covairates as possible into the model.
Tags:
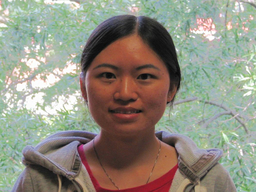