Graduate Students Seminar
Location
Mathematics/Psychology : 101
Date & Time
September 25, 2019, 11:00 am – 11:50 am
Description
Session Chair: | Ellie Gurvich |
Discussant: | Dr. Shen |
Speaker 1: Eswar Kammara
- Title
- A Distributed Algorithm for Dictionary Learning over Networks
- Abstract
- In this talk, we present a distributed algorithm for a nonconvex and nonsmooth dictionary learning problem proposed by Zhao et.al. The algorithm, named proximal primal-dual algorithm with increasing penalty (Prox-PDA-IP), is a primal-dual scheme, where the primal step minimizes certain approximation of the augmented Lagrangian of the problem, and the dual step performs an approximate dual ascent. We provide a proof outline for convergence to stationary points, which is mainly based on constructing a new potential function that is guaranteed to decrease after some finite number of iterations. Numerical results are presented to validate the effectiveness of the proposed algorithm.
Speaker 2: Zhou Feng
- Title
- Comparison of Causal Methods for Average Treatment Effect Estimation Allowing Covariate Measurement Error
- Abstract
- In observational studies, propensity score methods are widely used to estimate the average treatment effect (ATE). However, it is common in real world data that a covariate is measured with error, which violate the unconfoundedness assumption. Ignoring measurement error and using naïve propensity scores estimated by observed covariates will lead to biased ATE estimates. There are only a few causal methods that control the influence of covariate measurement error in ATE estimation, and there is no literature comparing their numerical performances. We conduct systematic simulation studies to compare the methods under rationales with respect to Gaussian vs. binary outcome, continuous vs. discrete underlying true covariate, small vs. large treatment effect, and small vs. large measurement error. The results show that under Gaussian outcome, bias correction method and latent propensity score method using EM algorithm perform best with small and large measurement error respectively; under binary outcome, the inverse probability weighting method and the latent propensity score method using MCMC algorithm perform best with small and large measurement error respectively.
Tags:
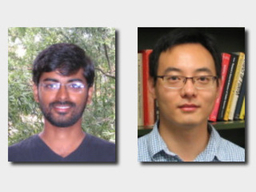