Graduate Students Seminar
Location
Fine Arts : 215
Date & Time
March 11, 2020, 11:00 am – 11:50 am
Description
Session Chair: | Carlos Barajas |
Discussant: | Dr. Rathinam |
Speaker 1: Sumaya Alzuhairy
- Title
- —
- Abstract
- We investigate the design and analysis of multilevel preconditioners for optimal control problems constrained by elliptic equations with stochastic coefficients. Assuming a generalized polynomial chaos expansion for the stochastic components, our approach uses a stochastic collocation on sparse grid for the PDE, thus leading to a discrete optimization problem. The stochastic collocation discretization technique requires many decoupled PDE solves to compute the gradient and Hessian. We show that the multilevel preconditioning technique from the optimal control of deterministic elliptic PDEs has a natural extension to the stochastic case and exhibits a similar optimal behavior with respect to the mesh size, namely the quality of the preconditioner increases with decreasing mesh-size at the optimal rate. Moreover, under certain assumptions, we show that the quality is robust also with respect the two additional parameters that influence the dimension of the problem radically: sparse grid dimension and stochastic dimension.
Speaker 2: Michael Lucagbo
- Title
- Combined One- and Two-sided Reference Regions for Multivariate Normal Measurements in Laboratory Medicine
- Abstract
- Reference regions are invaluable in the interpretation of results of biochemical and physiological tests of patients. Moreover, when there are multiple biochemical analytes measured from each subject, a multivariate reference region (MRR) is called for. Such reference regions are more desirable than multiple univariate reference regions because of their greater specificity against false positives. In some applications involving multivariate measurements, we may need two-sided reference limits for certain variables and one-sided reference limits for others. In this study, we develop an MRR that consists of a mix of one-sided and two-sided intervals for multivariate normal data. We compute the MRR as a prediction region. The approach uses the parametric bootstrap to estimate the prediction factors and hence construct the prediction region. The results show good coverage, even for small sample sizes and large dimensions.
Tags:
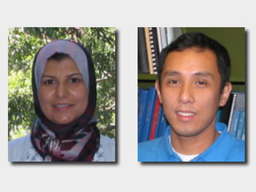