Doctoral Dissertation Defense: Zhou Feng
Advisor: Dr. Yi Huang
Location
Online
Date & Time
July 24, 2020, 8:00 am – 10:00 am
Description
Note: This dissertation defense will be conducted via WebEx
Abstract
Real-world evidence (RWE) is playing an increasing role in facilitating the development of innovative therapies and assessing the safety of post-marketing medical products. However, it is common that a covariate is measured with error in RWE studies, which violates the important unconfoundedness assumption in propensity score based causal inference framework. Ignoring such error and using standard propensity score approaches may lead to biased ATE (average treatment effect) estimate. To help approach selection, the performance of three causal approaches allowing covariate measurement error were compared by simulation studies under various scenarios, including different type of outcomes (Gaussian or binary), different type of error-free covariate (continuous or discrete), various sizes of ATE and various sizes of measurement error ranging from small to large. The results indicate that the bias correction approach is recommended under a Gaussian outcome and small measurement error, while the inverse probability weighting approach is recommended under a binary outcome and small measurement error; and the latent propensity score approach estimated by MCMC method is recommended under large measurement error for both Gaussian and binary outcome types.
Besides measurement error, another common issue in RWE studies is a non-directly observable outcome measured indirectly through multiple manifest outcomes. By extending our previous one outcome latent propensity score framework and adding a latent outcome piece, we proposed an innovative ATE estimation method using finite mixture model framework taking into account both covariate measurement error and non-directly observable outcome challenges. This newly proposed approach has at least two major advantages: First, it directly estimates the ATE on the true latent outcome. Second, it is flexible with different types of outcomes: The true latent outcome and any observed manifest outcome can be either Gaussian or binary. To evaluate the performance of the ATE estimation using newly proposed LPS method, we conducted simulation studies under various scenarios with respect to different type of true latent outcome, the various type of error-free covariate, various sizes of ATE and sizes of measurement error. All results indicate that the LPS approach works for all the scenarios, and in particularly works the best with smaller ATE and larger measurement error. Under a non-directly observable binary outcome, it is recommended to use risk difference as the ATE measure, followed by relative risk, and odds ratio should be the last choice.
Tags:
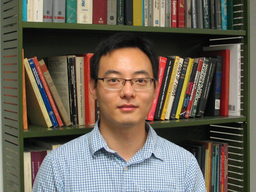