Statistics Colloquium : Dr. Minsuk Shin
University of South Carolina
Location
Mathematics/Psychology : WebEx
Date & Time
September 11, 2020, 11:00 am – 12:00 pm
Description
Title: Scalable Uncertainty Quantification via Generative Bootstrap Sampler
Abstract: A main goal of statistical analysis is uncertainty quantification in decision making, and the bootstrap procedures have been commonly used for this purpose. However, nowadays as the size of data massively increases and statistical models become more complicated, the implementation of bootstrapping turns out to be practically challenging due to its repetitive nature in computation. To overcome this issue, we propose a novel computational procedure called Generative Bootstrap Sampler (GBS), which constructs a generator function of bootstrap evaluations, and this function transforms the weights on the observed data points to the bootstrap distribution. The GBS is implemented by one single optimization, without repeatedly evaluating the optimizer of bootstrapped loss function as in standard bootstrapping procedures. As a result, the GBS is capable of reducing computational time of bootstrapping by hundreds of folds when the data size is massive. We show that the bootstrapped distribution evaluated by the GBS is asymptotically equivalent to the conventional counterpart, and empirically the approximation via the GBS is highly accurate. We examine the proposed idea to bootstrap various models such as linear regression, least absolute deviation regression, logistic regression, Gaussian mixture models, quantile regression, etc. The results show that the GBS procedure can not only accelerate the computational speed, but also attains a high level of accuracy to the target bootstrap distribution. Additionally, we apply this idea to accelerate the computation of other repetitive procedures such as bootstrapped cross-validation, tuning parameter selection, and permutation tests.
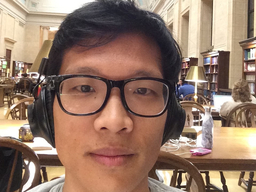