Graduate Students Seminar
On Blackboard Collaborate
Location
Online
Date & Time
October 13, 2021, 11:00 am – 12:00 pm
Description
Session Chair: | Gaurab Hore |
Discussant: | Dr. Yehenew Kifle |
Speaker 1: Nadeesri Wijekoon
- Title
- Godambe Information Matrix for Conditionally Specified Models
- Abstract
- In practice, Maximum Likelihood (ML) is the preferred estimation method of parametric models. However, if the likelihood function is complicated or contains an awkward normalizing constant, estimation using Maximum likelihood becomes difficult. Especially in conditionally specified distributions estimating using ML is difficult and computationally costly. Composite Likelihood (CL) is one of the approaches available to tackle this issue. Furthermore, due to the complexity of ML deriving the Fisher information is also becomes challenging. In this presentation, we will present an alternative approach for Fisher information called Godambe information which is based on CL. Further, we will briefly discuss how to derive the Godambe information matrix for conditionally specified models. Efficiency comparisons of maximum likelihood vs. composite likelihood will also be presented.
Speaker 2: Carlos Barajas
- Title
- Using Deep Learning to Improve Compton Camera Based Prompt Gamma Image Reconstruction for Proton Radiotherapy
- Abstract
- Proton beam radiotherapy is a method of cancer treatment that uses proton beams to irradiate cancerous tissue, while simultaneously sparing doses to healthy tissue. In order to optimize radiation doses to the tumor and ensure that healthy tissue is spared, many researchers have suggested verifying the treatment delivery through the use of real-time imaging. One promising method of real-time imaging is the use of a Compton camera, which can image prompt gamma rays that are emitted along the beam’s path through the patient. However, because of limitations in the Compton camera’s ability to detect prompt gammas, the reconstructed images are often noisy and unusable for verifying proton treatment delivery. Machine learning is able to automatically learn patterns that exist in numerical data, making it a promising method to analyze Compton camera data for the purpose of reducing noise in the reconstructed images. First, we provide motivation for training deep neural networks over standard ensemble techniques. We then present the usage of supervised deep neural networks to detect and exploit these patterns so that we can remove and correct the various problems that exist within our data.
Tags:
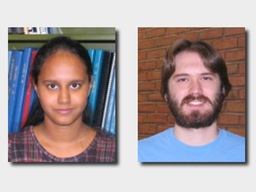