Doctoral Dissertation Defense: Reetam Majumder
Advisor: Dr. Nagaraj Neerchal
Location
Online
Doctoral Dissertation Defense: Reetam Majumder – Online Event
Date & Time
October 19, 2021, 4:00 pm – 6:00 pm
Description
Title: Hidden Markov Models for High Dimensional Data with Geostatistical Applications
Abstract
Stochastic precipitation generators (SPGs) are a class of statistical models which generate synthetic data that can simulate dry and wet rainfall stretches for long durations. Generated precipitation time series data are used in climate projections, impact assessment of extreme weather events, and water resource and agricultural management. In this thesis, we construct SPGs for daily precipitation data that is specified as a semi-continuous distribution with a point mass at zero for no precipitation and a mixture of Exponential or Gamma distributions for positive precipitation. Our generators are obtained as hidden Markov models (HMMs) where the underlying climate conditions form the states.
Maximum likelihood estimation of an HMM's parameters has historically relied on the Baum-Welch algorithm, which is a modification of the Expectation Maximization algorithm. We implement variational Bayes (VB) as an alternative estimation procedure for HMMs with semi-continuous emissions. Stochastic optimization in the form of stochastic variational Bayes (SVB) has been employed for computational speedup in practical cases. A univariate state process is often unable to adequately capture the underlying weather conditions over large watersheds, since different areas can have local weather regimes. We extend the HMM to a linked HMM (LHMM) where locations are divided into clusters. Each cluster's emissions are assumed to arise from a cluster-specific state process; the state processes are correlated and together form a multivariate Markov chain (MMC). The MMC provides more flexibility to accommodate heterogeneity that might be present in larger geographical areas. A Gaussian copula is constructed to capture the correlation structure of the MMC. Finally, we also construct a Gaussian copula for the emissions of the HMM to explicitly represent the pairwise correlations of observed positive precipitation. Daily precipitation data over the Chesapeake Bay watershed in the Eastern coast of the USA is used as a demonstrative case study. Remote sensing precipitation data is sourced from the GPM-IMERG dataset for the wet season between July to September from 2000-2019. Synthetic data generated from the clustered LHMM with can reproduce the monthly precipitation statistics as well as the spatial correlations present in the historical GPM-IMERG data.
Tags:
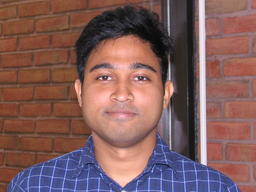